Spotlights
[Research News] Enhancing Automatic Image Cropping Models with Advanced Adversarial Techniques
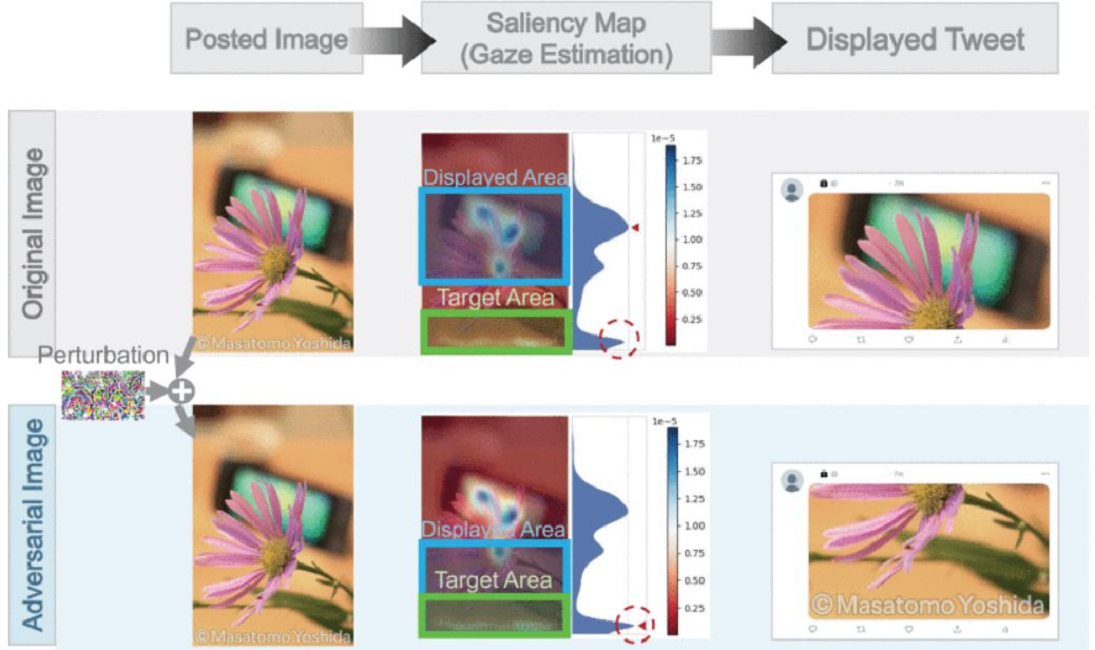
Many commercial image cropping models utilize saliency maps (also known as gaze estimation) to identify the most critical areas within an image. In this study, researchers developed innovative techniques to introduce imperceptible noisy perturbations into images, thus influencing the output of cropping models. This approach aims to prevent essential parts of images, such as copyright information or watermarks, from being inadvertently cropped, thus promoting fairness in AI models. .
Researchers, including Masatomo Yoshida, a Ph.D. student at the Graduate School of Science and Engineering, techniques to create perturbations in images that can deceive cropping models, helping identify and address their weaknesses.
Models that automatically crop images, like those used in social media to create thumbnails, can sometimes make mistakes, leading to misrepresentation. To study and address these models' weaknesses, they have developed novel methods for creating adversarial examples. By efficiently generating adversarial images that modify actual images to 'trick' models into cropping different regions, these techniques aim to make image cropping AI systems more reliable and fair.
Reference
M. Yoshida, H. Namura and M. Okuda, "Adversarial Examples for Image Cropping: Gradient-Based and Bayesian-Optimized Approaches for Effective Adversarial Attack," in IEEE Access, vol. 12, pp. 86541-86552, 2024.
https://doi.org/10.1109/ACCESS.2024.3415356
This achievement has also been featured in the “EurekAlert!.”
NEWS RELEASE 1-AUG-2024, Enhancing Automatic Image Cropping Models with Advanced Adversarial Techniques
Image Credit: Masatomo Yoshida from Doshisha University
License type: Original content
Contact |
Department of Research Planning PHONE:+81-774-65-8256 |
---|
Category
- Doshisha University Official Website:
- Top Page /Research /Current Student /Alumni Student /